The objective of the report is to conduct a technological intelligence on “Medical Imaging.” The Medical Imaging report includes the following aspects:
- Recent technical advancements including key innovations and innovative trends
- Market research covering market size, market dynamics, and market segmentation
CT scans acquire a major share in medical imaging followed by X-rays, MRI, and then nuclear medicine. Recent innovations have used machine learning algorithms to automatically segment blood vessels in MRI images. The trained neural networks and deep learning networks are used to suppress the motion artifacts.
Challenges
- Currently in CT images when lung nodules are detected, the lung structure is not segmented and false positive results in lung nodule detection are high.
- Motion artifacts become challenging when patients are unable to hold their breath due to hiccups and coughing.
- Manual regionalization of blood vessels is a time-consuming process as it takes a long time to localize blood vessels, and errors may occur due to mistakes of an operator, and the same result for each operator for the same 3D medical image.
- The handling of multiple patient images in cancer treatment is a tedious process.
- The iterative reconstruction method has a long calculation time and is difficult to apply in practice.
Era of Digitalization
This section of the Medical Imaging Report focuses on the significant advancements and research conducted on computed tomography based on artifacts, modality, limbs, and other factors.
Lung nodule screening based on deep learning technology
Image preprocessing is carried out in a series of processes including an image analysis program, an image filtering process, target sub-image acquisition, and histogram equalization processing.
This lung nodule screening method based on deep learning technology uses 2D space for performing edge completion through the morphological operations in accordance with the concave-convex property of the segmentation contour. It prevents nodules on the lung wall from going overlooked. And 3D information of the focus on the CT sequence image is fully utilized to effectively identify the type of nodule.
Automatic segmentation of vessels by ML image processing algorithm
This method and system automatically segmentize the blood vessels of a medical image using machine learning and image processing algorithms. It uses a region-growing algorithm for a blood vessel region of the input 3D image data using coordinate information of the generated 3D shape machine-learning data.
AI-based image analysis for detection and characterization of lesions
Improved detection and characterization of lesions within an individual using automated analysis of nuclear medical images. It involves Positron Emission Tomography (PET) and Single Photon Emission Computed Tomography (SPECT) images.
The machine learning module is a region-specific module that receives, as input, a specific portion of the 3D functional image corresponding to one or more specific tissue regions and/or anatomical regions of the subject.
Metal artifact removing method & system for oral cavity cone beam CT image
This system removes the metal artifacts in an oral cavity cone beam CT image. The original projection sinogram is processed to carry out several processes including FDK reconstruction, metal region segmentation & extraction, then Linear interpolation correction is performed which removes the streak artifacts but the structural information is lost. Therefore, it reconstructs the original image by backfilling metal portions into the de-artifact reconstructed image to obtain a final image without the metal artifacts.
Motion artifacts suppression with computed tomography imaging
Instead of motion artifact reduction in X-ray imaging, this system arranges the images to a computer program element in a computer-readable medium.
The operation of the motion analyzer is based on forming a difference between the two input images and analyzing the difference between the values. The input images are de-noised first to analyze further by the motion analyzer to establish robust motion estimates.
Each year Signicent provides consultancy to hundreds of organizations to help transform their innovations to value.
Market
According to the research by the market experts of Signicent, the global medical imaging market is expected to grow at a CAGR of 5.8 % during the timeline of 2021 to 2028. It was valued at USD 37.97 Bn in 2021 and is anticipated to reach the value of USD 56.53 Bn by 2028.
Market Segmentation
Based on Technology, the market can be divided into the following segments.
- X-ray
- Nuclear Imaging
- MRI Scan
- CT Scan
Key Players
This section of the medical imaging report highlights the key players actively working in the healthcare industry.
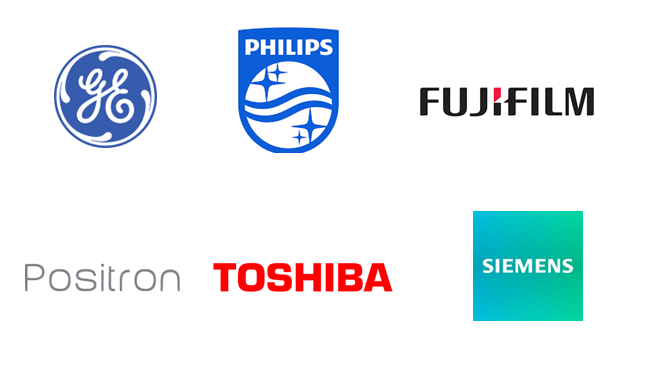
Recent Report
- AI in Biotechnology: Transforming Medicine, Food, and Animal Health
- The future of AI in Automotive Industry
- AI in Beauty and Personal Care: Transforming Skincare, Fragrance and Modeling
- Bioplastics in Healthcare – Sustainable Plastics for a Greener Future
- Sustainable and Natural Flavor Production with Biotechnology